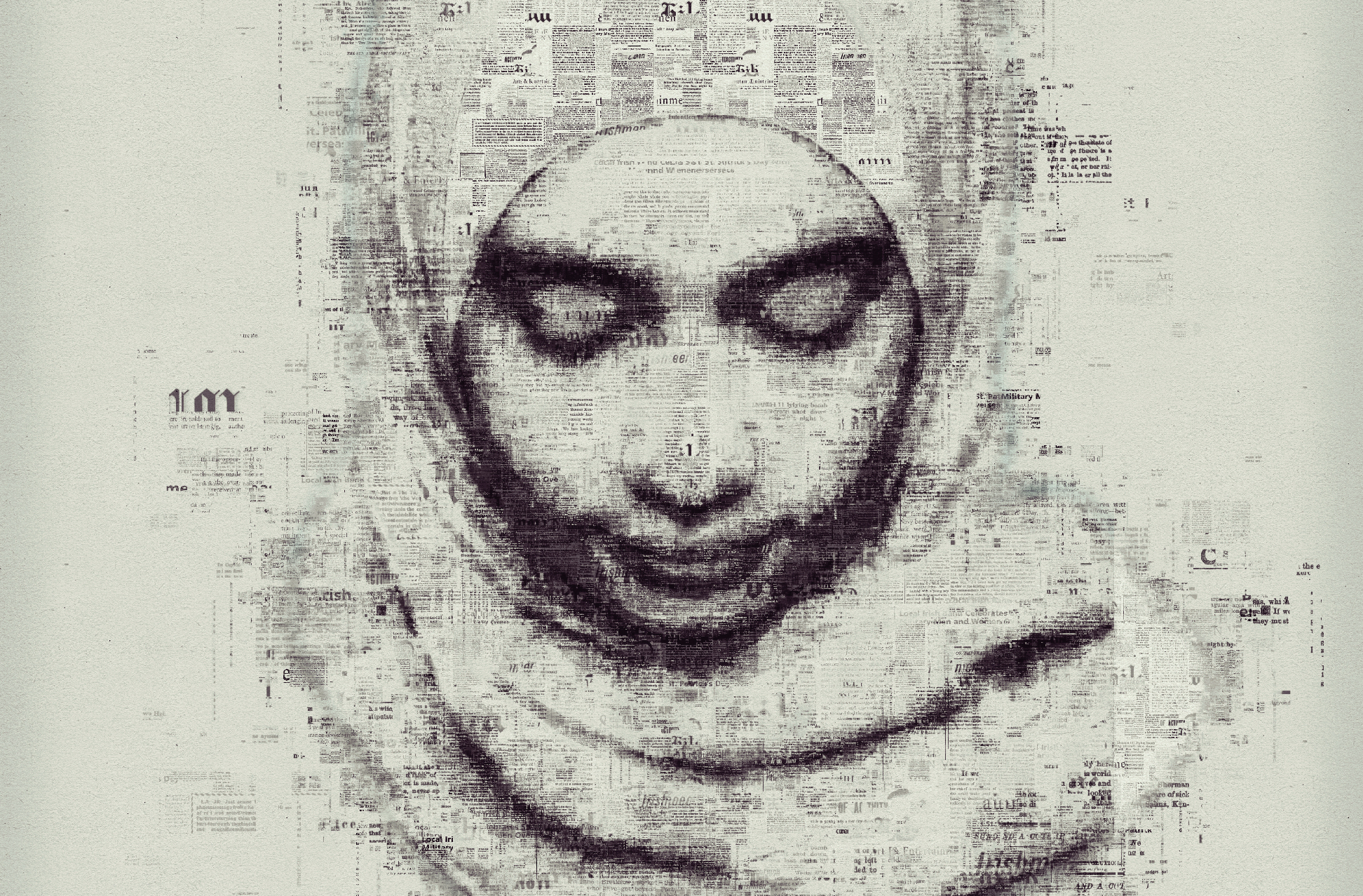
Rooting Out the Artificial in Artificial Intelligence
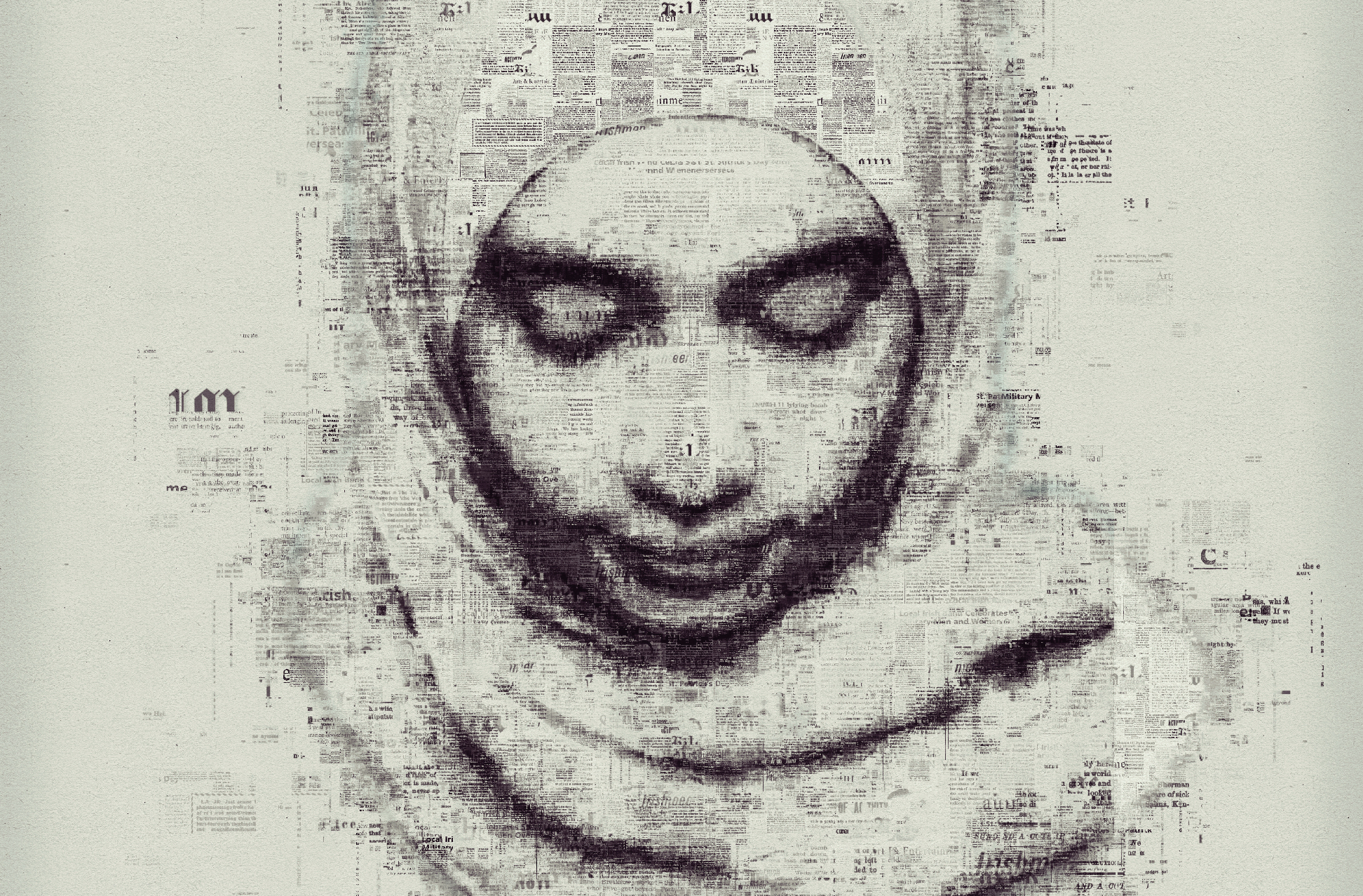
In May 2021, Lemonade, a provider of homeowners, rental, and pet insurance, extolled in a tweet the ability of its facial recognition software algorithm to accurately detect claims fraud: “When a user files a claim, they record a video on their phone and explain what happened. Our AI carefully analyzes these videos for signs of fraud. It can pick up non-verbal cues that traditional insurers can’t, since they don’t use a digital claims process.”
Potentially biased AI algorithms in underwriting, pricing and claims processes have led to a growing chorus of criticism.
The use of AI models by insurers is under intense scrutiny by the media, the federal government, congressional leaders, lawyers, and regulators.
If the algorithms used to speed up certain tasks are embedded with biased data, the information modeled is worthless. Or worse.
The declaration that an algorithm could “pick up non-verbal cues” to discern “signs of fraud” ignited a firestorm of criticism. Many people assumed that insurers evaluated claims based on objective data such as financial information and photographs. Instead, they were making this decision based in part on how a person looks.
The tweet went viral, attracting widespread media attention. As Vox magazine reported, “The prospect of being judged by AI for something as important as an insurance claim is alarming to many who saw the threat, and it should be. We’ve seen how AI can discriminate against certain races, genders, economic classes, and disabilities, among other categories, leading to those people being denied housing, jobs, education, or justice.”
Lemonade quickly deleted the tweet and subsequently retweeted that the use of the phrase “non-verbal cues” was “poorly worded,” explaining that its facial recognition software flagged suspicious claims that were sent to claims specialists for analysis. The software did not automatically reject a claim the algorithm suggested was fraudulent.
By that point, the industry was on the defensive. The resulting outcry added to a growing chorus of criticism over potentially biased AI algorithms in underwriting, pricing and claims processes. AI algorithms were touted for making the purchase of insurance simpler, the processing of insurance more efficient, the coverage of risks more comprehensive and the cost of insurance less. But these cutting-edge technologies appeared to be doing a disservice to an increasingly diverse society.
Virtually overnight, the use of AI models by insurers was under intense scrutiny—not just by the media but also by federal government agencies, congressional leaders, state insurance regulators and litigation-seeking law firms. The interest by the legal profession is sobering, of course. No insurer wants to be hit with a class-action lawsuit alleging discriminatory practices. Yet it appears that plaintiff law firms are sharpening their knives in anticipation, as this blog on the website of insurance attorneys Raizner Slania suggests:
It is likely that Lemonade’s system, whether purposeful or not, has a propensity for racial profiling when evaluating customers’ videos detailing their claims as it relies on information other than objective data of the actual damage, such as facial expressions and physical appearance. Evaluating claims based on non-objective data, or “non-verbal cues,” such as racial or ethnic data, accents, genders, age, etc., leads to a real risk of wrongfully denying claims through racial profiling.… There is a real question of legality as claims may be denied based on no reasonable basis.
This risk now must be weighed against the many upsides of AI models. Algorithms can analyze mountains of structured and unstructured data to speed up underwriting, pricing and claims tasks. If the algorithms are embedded with unintentionally biased data, the information modeled is worthless. Or worse. An insurer’s reputation for fair dealing is at stake.
Causes of Bias
The use of non-verbal cues is just one instance of an AI model using questionable data. Using old data is another major cause of biased information creeping into an AI model. Data that reflect a historical bias or prejudice can be used to train the machine learning algorithms that guide the outcome. Such biases continue to thrive unbeknownst to the user, perpetuating over time to contaminate future AI models.
Reuters reported that Amazon used historical data in training its AI model for recruitment possibilities. The information was 10 years old. Since men dominated employment ranks in the tech industry at the time, the machine-learning model was incorrectly trained to conclude that male job candidates were preferable over female candidates. Like Lemonade, Amazon responded that its hiring decisions were not based entirely on the AI model’s recommendations. The company subsequently edited the AI model to make it gender neutral.
Facial recognition algorithms using old data sets also tend to skew primarily to white men, resulting in a lower accuracy rate in correctly identifying the faces of people of color, women, children and gender-nonconforming people.
Old sources of data used to underwrite automobile insurance often include geographic ZIP codes correlating with a particular race, increasing the possibility of biased decisions involving people with different ethnic backgrounds.
These examples also demonstrate what happens generally if the AI model is not fed enough data to make a balanced decision. An imbalance can occur if the algorithm is fed more data on men than women, white people than black people, straight people than LGBTQ people, older people than younger people, and so on.
Shameek Kundu, head of financial services and chief strategy officer at TruEra, a provider of AI quality solutions, offers the example of a banking client that modeled its credit approval data across men and women. “This is an area where women are known to be more creditworthy than men, and yet the model predicted women to have lower credit approvals,” he says. “The reason is there were nine men for every woman in the data points. This created an imbalance that skewed the results.”
Another factor in biased data has to do with the cognitive biases of the designers creating the algorithm. Every human being has implicit assumptions and unconscious biases about other people; these built-in stereotypes affect our decisions.
Yet another potential for bias derives from machine-learning models trained to translate language meanings. Female and male names, for example, may be interpreted as gender tropes. For example, words such as nurturing and productive often align, respectively, to women and men, thus affecting career prospects. A company looking for a “more productive sales leader” may be guided towards predominantly male candidates.
Unscrambling an Egg
Despite all of the unintended consequences of artificial intelligence, many agree that AI tools are still worth using. AI tools that “crunch data and spit out answers about tendencies, probabilities and predictions at extraordinary speeds” are too valuable for insurance companies and other businesses to ignore, says Lee Rainie, director of internet and technology research at the Pew Research Center. (Rainie’s comments are drawn from subject matter experts and are not his own opinions.)
“People can do the same thing, peering into thousands of rows and columns in spreadsheets,” Rainie says, “but that takes inordinate time to reach conclusions.”
Like others interviewed for this article, Rainie says insurers are not purposefully trying to discriminate against protected classes. “We’re told there are no malevolent programmers trying to figure out how to hurt people; there are just blind spots in the data,” he says. “The challenge for insurers is to find the ways that bias creeps into their AI calculations.”
Finding the bias in their models, however, will not be easy. “Unfortunately, the AI process is undermined by its opacity,” says economist Robert Hartwig, a professor of finance and director of the Risk and Uncertainty Management Center at the University of South Carolina.
In using AI and machine learning tools, billions of data points are fed into an algorithm that is trained to rapidly refine millions of people into a population of one, Hartwig explains. “The problem is, as the algorithm becomes increasingly complex, it transforms into a ‘black box’ whose internal data is so vast it is essentially unknown. Consequently, regulators can’t see into the box to determine how an individual came to be charged what they were charged for an insurance product.
“Once the algorithm is fed billions of data points, you can’t just pull them out one by one to determine if a single data point is flawed from the standpoint of bias,” Hartwig says.
While he believes insurers will be successful in eliminating some biases by using machine learning tools trained to search for instances of bias, “They can’t eliminate them all, because the architecture of the algorithm makes it impossible,” Hartwig asserts. “It would be the equivalent of unscrambling an egg.”
Others, however, disagree. TruEra is predicated on digging out these instances of potential bias and debugging AI models of their presence. Launched in August 2020, the company has created a suite of AI-quality diagnostic solutions that open the “black box,” making it transparent to data scientists and other model developers. The diagnostics analyze why an AI model makes specific predictions, ensuring accuracy, reliability, and fairness, TruEra’s website states.
More recently, the company unveiled a new solution that continuously monitors machine-learning models, pinpointing new sources of data that may be biased or erroneous. The customer is subsequently alerted to a potential instability in its AI model. If requested, TruEra will then exterminate the bug. “The insurance industry is a major focus for us,” Kundu says.
In that regard, the company has retained David Marock, former CEO at Charles Taylor, a global insurance services and technology company with 120 offices across 30 countries, as a senior advisor. “TruEra can help insurers overcome the challenges of black box bias through much better transparency,” says Marock, who also is a senior advisor at McKinsey & Co. “For the insurance industry at the moment, there is a crucial need to demystify AI to win the trust of the public and regulators.”
Rainie believes that finding new ways to build models without bias and then putting the regulations in place to protect consumers against bad models would be a start. “One idea is for the creators of AI models to be a diverse group of individuals,” he says, “which would minimize the blind spots by having a mix of people from different races, genders and socioeconomic circumstances conceptualizing this stuff.”
He cites recommendations for an AI algorithm audit and an enforcement regime—the establishment of consumer protection laws requiring that AI algorithms and models are structured to ensure the needs of all people are first and foremost in users’ minds.
AI Use Under Review
Ultimately, these are important conversations to have. Just as the use of AI algorithms in financial services has grown, so has the U.S. government’s interest. These events have all occurred just this year.
- In February, a group of U.S. senators called upon the Equal Employment Opportunity Commission to investigate bias in AI-driven insurance pricing, lending, advertising and hiring decisions.
- In May, hearings were held by the U.S. House of Representatives Task Force on Artificial Intelligence on “How Human-Centered AI Can Address Systemic Racism and Racial Justice in Housing and Financial Services.”
- In June, President Biden launched the National Artificial Intelligence Research Resource Task Force to guide the development of AI systems across public and private sectors.
- In June, the National Institute of Standards and Technology (NIST) issued a proposal to identify the possibility of bias in AI algorithms.
“The proliferation of modeling and predictive approaches based on data-driven and machine learning techniques has helped to expose various social biases baked into real-world systems, and there is increasing evidence that the general public has concerns about the risks of AI to society,” the NIST proposal stated.
Earlier this year, the National Association of Insurance Commissioners created the Big Data and Artificial Intelligence Working Group. The group’s mission is to “evaluate insurers’ use of consumer and non-insurance data and models using intelligent algorithms, including AI,” the association stated. Iowa State Insurance Commissioner Douglas Ommen, the working group’s chair, says the group is in an information-gathering stage.
“An information letter went out to property and casualty carriers on Aug. 4 asking about possible issues associated with external bias in automobile insurance underwriting, although we will eventually touch on other lines,” Ommen says. “Every state is grappling with this issue as insurers use these new technologies.”
Whether insurers will be required to prove the AI algorithms used to model risks are unbiased is not on the table at this point. “The information gathered from insurers will be confidential to assist regulators in where we need to focus,” Ommen explains. “Once this work is completed, my hope is that carriers will have auditing systems in place to test for external bias, demonstrating that [underwriting] is exclusively tied to risk and is not influenced by measures associated with issues like race, which is unlawful. We can then be in a position to better describe to the public and other interested parties what we expect of the insurers.”
Verisk Analytics is one of the world’s top data analytics and risk assessment firms, a public company whose IPO in 2009 was the biggest of the year, providing a windfall to its former insurance company owners. Since that time, the firm has acquired multiple technology companies that expand its advanced analytics capabilities.
Via these transactions and its own capabilities, Verisk leverages an array of sophisticated technologies like machine learning, neural networks, natural language processing and image recognition software to help its insurance industry clients model risk and fraud in their underwriting, pricing and claims practices. Such practices in the insurance sector have been widely criticized for guiding potentially discriminatory decisions, due to data that may be biased against protected classes of people.
“This issue is at the forefront for us today,” says Jim Hulett, vice president of product innovation at Verisk Analytics. “We take the risk of bias in the data our products analyze very seriously. There is data out there we simply will not touch with a 10-foot pole. We also don’t deal in ‘black box’ solutions.”
Verisk takes pains to ensure the data its AI models interpret are free of bias, Hulett says. “We’ve instituted a formalized peer-review process to screen data on a holistic basis for evidence of possible bias, a task that we entrust to a team of data scientists, legal experts and compliance leaders,” he says. “Anything that is considered biased from the standpoint of race, gender, and other factors is carefully vetted and, if deemed a concern, is broadly shared across the organization. We also make sure whoever is using our solutions is provided support for the predictions provided.”
He offered the example of the firm’s claims fraud solution, which models potential fraud propensity—highly questionable patterns in a claim that align with proven fraud cases.
“We point out these dynamics to the customer,” Hulett says. “It is up to them to send a questionable claim to their special investigative unit (SIU) or a specialist claims adjuster for further analysis of fraud. If the analytics suggest a possible fraud incident, it’s up to claims adjusters and SIU investigators to determine if this is indeed the case. This human touch is crucial, we tell clients.
“All technology does is get the right work to the right people quicker. It’s their obligation to take it from there.”