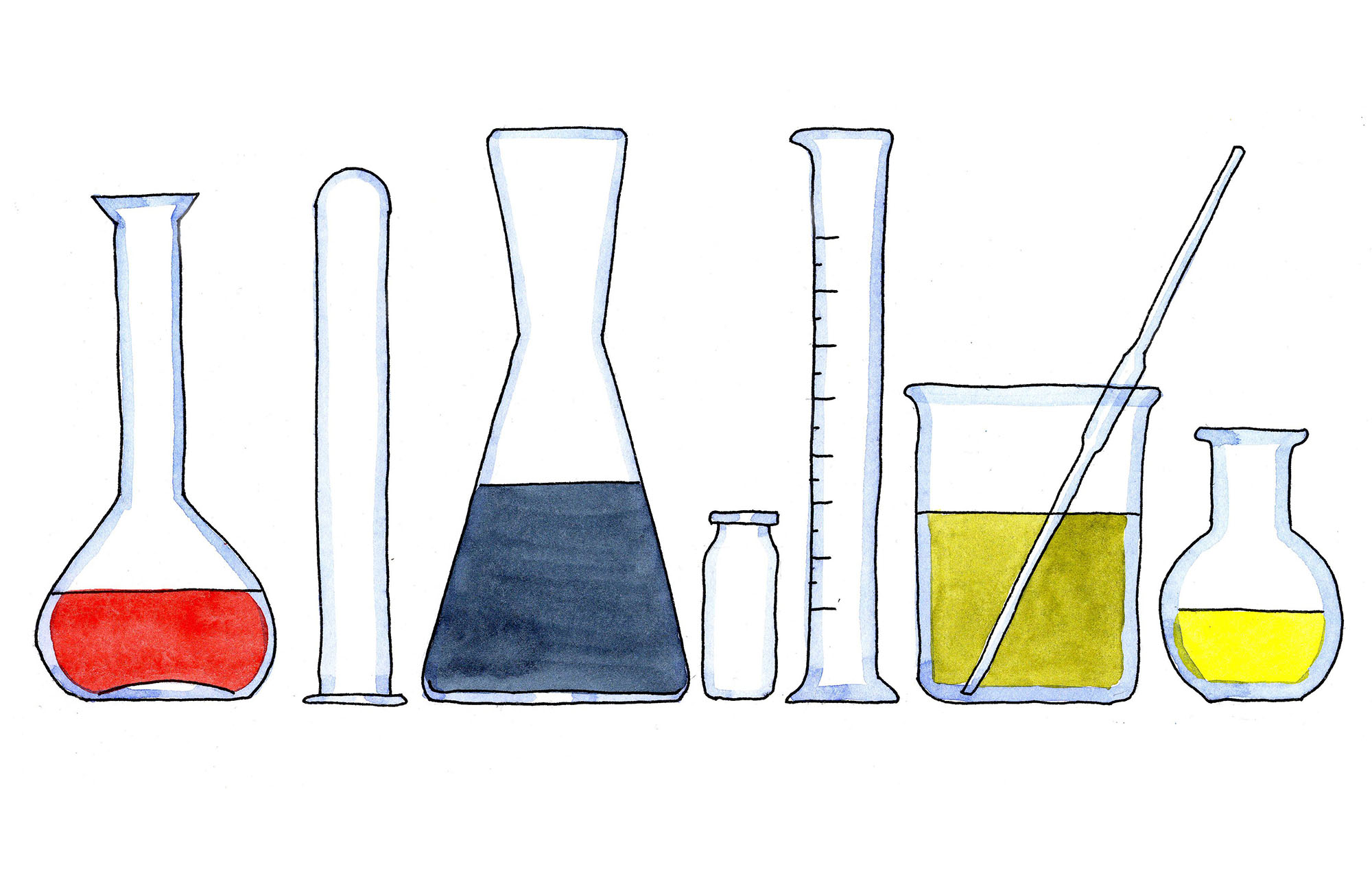
Risk in a Test Tube
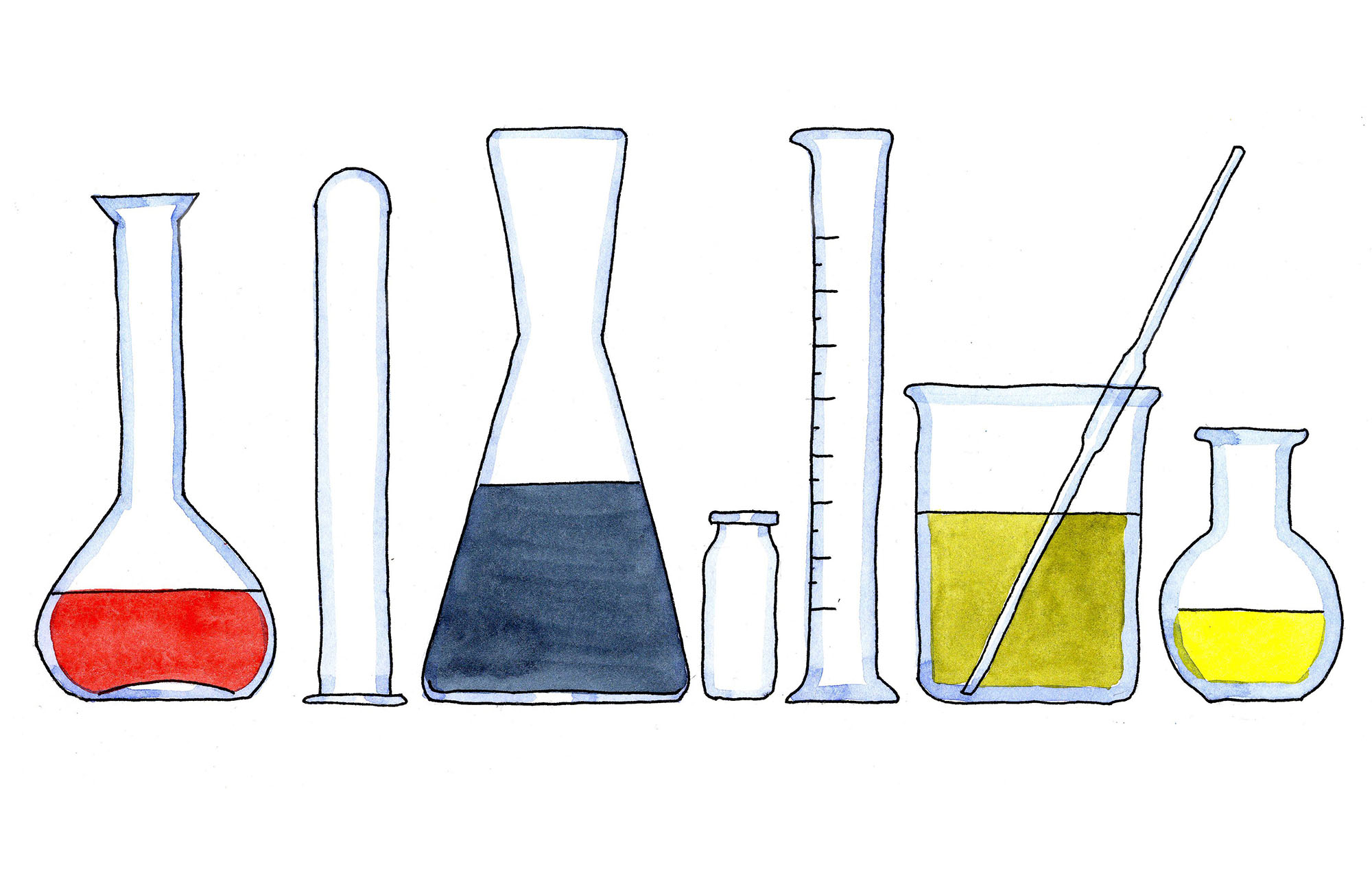
Nicholas Barbon, an English physician, economist, property developer, and insurance man, explained the science of underwriting in 1684.
The entrepreneur, who had launched two insurance companies (and according to the Insurance Hall of Fame “deserves the title Father of Fire Insurance”) imagined a town with 300 insured brick houses each worth an average of £100 (it was a long time ago) and a total loss frequency of 10 per year (it was a dangerous time).
“In one Month Four Houses are Burnt, which Were Insured at Three Hundred Pounds,” Barbon wrote, “So that by Twenty Shillings [£1] a House, among Three Hundred, the Loss is paid.” His analysis continues with various frequencies and sums insured until he concludes that “in Seven Years and a Half, a fourth part of the Town is Burnt and Rebuilt…every man hath equally Lost a Fourth part of his House: Those that were Not Burnt, by paying the Premiums, to those that Were Burnt…there is no Difference between the Insured & Not Insured. Betwixt those that Were Burnt; And Not Burnt.”
His probabilistic calculations are hardly actuarial science, but the basis of calculating premiums isn’t really very different today. They took a step forward in the 1970s with the injection of computing power, when Nick Thomson, reinsurance underwriter of the Hiscox syndicate at Lloyd’s, created a computerized catastrophe risk pricing engine. He developed a methodology for pricing excess of loss reinsurance using an Epson calculator programed with his own catastrophe algorithms. The results he produced were at least credible—and soon influential. The science of underwriting had taken another step forward.
Data fueled underwriting science in the 17th and 20th centuries, and insurance today is typically even more data-intensive. Matthew Fosh, executive chairman of the tech-driven London MGA Optio, recently told a public forum, “We are becoming a data business.” In the same Insurance Day panel discussion, Jason Howard, CEO of the recently re-branded brokerage Acrisure Re (formerly Beach & Associates) said his firm is collecting “hundreds of datapoints on ten million clients,” adding that “data is a fundamental starting point for any conversation.”
Data remain the fuel that fires the science, the gas in the Bunsen burner. As if to prove the point, a December survey of insurers by State Street found that 91% of sector personnel face increased complexity in their organization’s use of data to improve their operating models and infrastructure.
Algorithms which would have astounded Barbon today almost exclusively price auto and homeowners risks. Small business cover is typically rated the same way, and the practice is creeping, beneficially, into the middle market. Even Lloyd’s, the bastion of underwriter instinct, is beginning to embrace the science. Lloyd’s carrier Brit Insurance claims to be “Writing the Future” with its launch for 2021 of Ki, a follow-only syndicate that Brit describes as “the first fully digital and algorithmically-driven Lloyd’s of London syndicate that will be accessible anywhere, at any time.” Ki is linked to Google Cloud and aims to “redefine the commercial insurance market.”
The science of underwriting is all very well and good—and significantly cheaper to deploy—when the data are rich and available. When they aren’t, though, the science gets slippery. Much has been made, for example, of advances in modeling the blast damage that would occur if a terrorist were to explode a bomb in an urban center. The science of computational fluid dynamics has been used to estimate with serious confidence the likely impact on buildings located at various distances from the point of a detonation, given the other buildings that lie in its path. But ask terrorism risk modelers about loss frequency, and they will tell you they’re still working on it.
Specialty risks from terrorism to cyber to D&O have evolved quickly and changed dramatically, so quickly that it is very difficult to deploy the ergodic principle, a theory from economics which assumes the future will play out much as the past did. Even when the data are rich, we like to believe that history repeats itself, but the truth is that each incarnation is more like a third-generation photocopy than a photograph, simply because things change (driven by specters like social inflation, climate change, and political whimsy). Change makes application of predictive science much less feasible, but it doesn’t rule out a scientific approach entirely.
The challenge, it seems, is to get the balance correct. My insurance-journalism mentor, Trevor Petch of the long-lost Financial Times World Insurance Report, once told me that a specific syndicate at Lloyd’s was part of the “kamikaze contingent.” They’re the underwriters who lead the market up as well as down and embrace the art of underwriting to the complete exclusion of science. Their nil-science approach generated soft-market pricing decisions that were simply unsustainable and brought concentrated losses. The KamCon still exists and is now pricing to the opposite extreme. Today some are charging hard-market prices that could not be justified by even Barbon’s rudimentary scientific approach.
It is near impossible, of course, to use science to support pricing decisions when data are limited or the ergodic principle has exploded after external forces have driven a significant change in frequency, severity or both. But an approach levering more than just guesswork and competitive inertia is surely appropriate. To that end, Lloyd’s has embarked on a controversial effort to tame the still-sizable KamCon through the introduction of underwriting related “minimum standards” aimed at injecting at least a little science marketwide.
Lloyd’s “Minimum Standards MS3 – Price and Rate Monitoring,” dated January 2021 but published on its website earlier, promises “a new Best Practice Pricing Framework (BPPF)…designed to give Lloyd’s and its key stakeholders confidence that syndicates have in place appropriate, proportionate and well understood pricing processes which are being used in key decision making…with an aim of raising market standards across technical pricing, and portfolio management.”
The BPPF has yet to be published, but MS3 includes plenty of prescriptive underwriting science without it. Henceforth Lloyd’s will explicitly require that “pricing is informed by experience and exposure data (if available/reliable), and not only by benchmarks and expert judgement, within a structured process or model” and that “the pricing of excess of loss business should be supported by proven actuarial techniques and, where appropriate, reflect ground-up experience and exposures for a given programme (if available), and not only the historical experience of the excess layer.” Space prohibits inclusion of the many more examples of Lloyd’s emerging science of underwriting.
More than a handful of Lloyd’s practitioners are unhappy with this central interference in their approach to pricing risks. It’s akin to the Spitalfields Market telling its stallholders how to price a bushel of apples. To them it feels like the authorities on the top floors of One Lime Street intend to run Lloyd’s more like an insurance company than a market comprising individual insurance businesses. However, if Lloyd’s is to be as profitable as possible, all its underwriters need to deploy at least a little science where possible—without quashing the market’s famous appetite to insure almost any conceivable risk, regardless of the data.