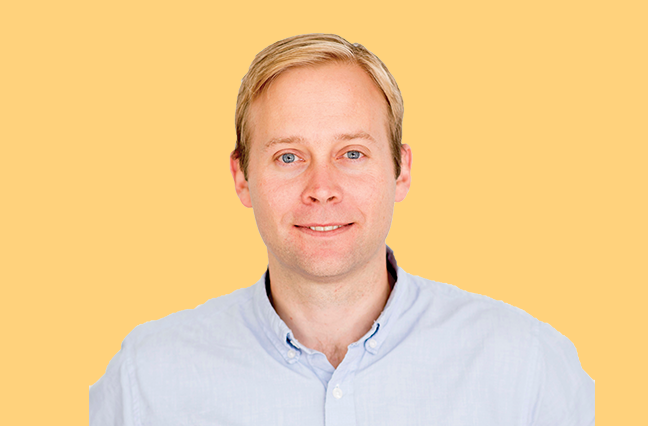
Machines Learn Policy Review
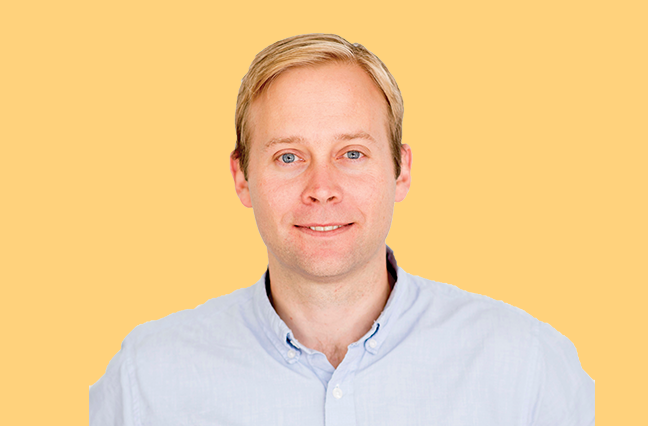
As he pored over millions of documents, Chris Cheatham decided there had to be a better way. His startup, RiskGenius, applies machine learning to speed policy review.
Like any good entrepreneur, I said, “Sure! What’s policy review?” I really didn’t know a whole lot about policy review at that time. Slowly but surely, we realized that this was a much bigger problem than the claim document problem we were solving and that we should go solve this policy review problem.
Insurance language could be a clause that excludes any damage by war or terrorism or government. Another clause might say, “We do not accept responsibility for anything damaged if there is an attack, which involves missiles by a government person.” Those two different clauses may essentially mean the same thing.
The first thing we did on machine learning was we trained our machine learning platform—we call it Johannes—to understand that both clauses mean the same thing. Both should be labeled “Exclusion—War.” We’ve done this work on more than a million clauses now and identified about 3,000 categories. As of now, we can take wording from one carrier and wording from another carrier and line up similar clauses, even though these clauses are written differently.
Step two was to go deeper into the policy and not only pull out the policy language but also pull out the numerical values.
Imagine you have a war policy deductible for $10,000 and on another policy you have a deductible for a missile shot by a government person and that deductible is for $100,000. In the end, the broker needs to be able to line those up and tell their clients that both of these are deductibles for war and this one’s for $10,000 and this one’s for $100,000 and advise them which one’s better. In that case, you have to be able to apply a common label across both deductibles and show how the data compare. That’s our Policy Check tool we recently launched. It’s standardizing the language and the numerical values no matter what an insurance policy calls it.
On the broker side, there is a lot of demand for the best insurance product possible. A lot of time brokers don’t have the time to provide that analysis to their customers, particularly small and medium-sized customers. Brokers can’t put in the time needed to do that type of work because they need to focus on the big accounts. Our goal is to free up the brokers to do more policy analysis faster so they’re doing less of that repetitive work. In the long run, it’s going to create better relationships between brokers and their customers because they’re going to spend more time on risk management and finding what’s in the customer’s policy and what the best coverage is.
Bytes
$697 million
Insurtech funding in Q4 2017
157%
Increase in funding from $271 million in Q4 2016
53
Total Q4 2017 transactions, second-highest quarter on record
41%
U.S. share of Q4 transactions, compared with an average of 62% since 2012
17%
U.K. share of transactions
64%
Share of Q4 investment rounds focused on front-end processes (product and distribution)
36%
Share of Q4 investment rounds focused on back-end processes
7
$30 million-plus funding rounds in Q4 2017
5
Half the top 10 Q4 funding rounds went to companies outside the United States
Source: Quarterly InsurTech Briefing from Willis Towers Watson and CB Insights