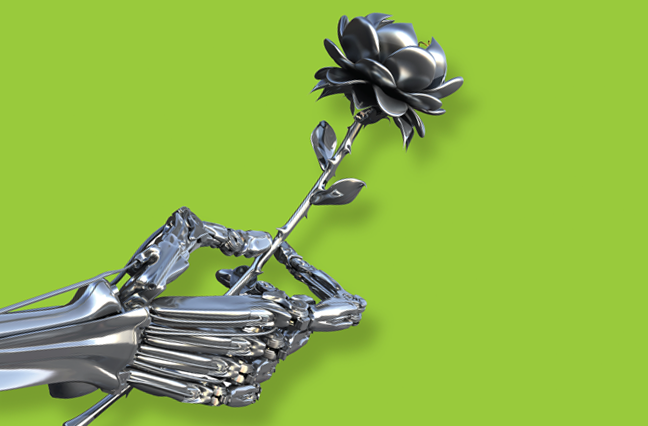
Almost Human
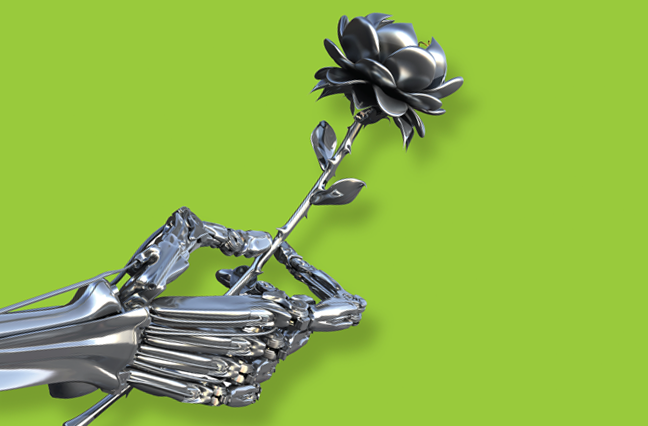
As we age, we feel we’ve acquired wisdom from life’s varied experiences. Why not the same for a machine?
Machine learning offers the means for insurance carriers and brokerages to augment the knowledge needed to make sharp underwriting, claims management, customer service and other profit-making decisions. This form of artificial intelligence is designed to recognize patterns that otherwise would escape the attention of mere human mortals. Like people, the machines become increasingly intelligent over time, albeit much, much faster.
Computers already transcend human intelligence and, in another generation or two, will be trillions of times more powerful than they are today. IBM’s computer Watson is a case in point. The system was built specifically to beat winning contestants on the quiz show “Jeopardy!” though its broader goal was to create a new generation of technology that can maximize the utility of unstructured data, IBM says. With access to more than 200 million pages of structured and unstructured content, including the full text of Wikipedia, Watson easily overcame the competition.
Now IBM Watson is assisting global insurer and reinsurer Swiss Re to increase its life and health reinsurance profits through a more informed understanding of potential loss exposures. Swiss Re just announced a partnership with IBM Watson to develop cognitive solutions that help underwriters accurately price risk.
Other insurers and brokerages have also latched onto machine learning. Chubb, for example, is applying machine learning to its workers compensation claims. The goal is to close claims faster and at less expense, while improving an employee’s medical outcome for a speedier return to work. Brokerages like Willis Towers Watson and Aon are using machine learning to better understand their clients’ loss drivers and related insurance coverage needs.
These organizations have come to realize that artificial intelligence can augment basic human intelligence. “No human being has the time or ability to look through millions of data sets to make sense of it for insurance underwriting or competitive purposes,” says John Franzis, the insurance industry leader at IBM Watson Group, which is dedicated to developing and commercializing cloud-delivered cognitive innovations. “There is a finite capacity to access the right information, understand it and make use of it.”
Ready, Set, Go
The industry is still at the beginning of the beginning in this embrace of machine learning, which is a technique used in predictive data analytics. Although the insurance sector has a long history of analyzing internal company data to underwrite and price products, other industries have led in the more robust use of predictive analytics.
Like insurers, these businesses are accessing internal data for analytical purposes, but they have added external data and unstructured data to the stew. Using brilliantly constructed algorithms, they are able to sift through millions of external data sets to unearth kernels of wisdom improving their decision-making. For example, the analytics may predict a decline in customer demand in a particular region in the next quarter of the year. Armed with this insight, the company can alter the pricing on a product and how it is advertised.
They are also using tools to analyze more than just structured data—the information that typically resides in a database or on traditional line-item spreadsheets. Such unstructured data could be written notes, texts, video and even voice memos. All of it is food for thought.
“I’ve been in and around the industry for 34 years, and the problem has never been a lack of information,” Franzis says. “The problem has been that a large portion of the information analyzed by the industry is internal and structured. The real information is unstructured. For example, a claim adjuster’s notes can be a treasure trove of insights, assuming there is a simple way to collect and analyze the notes.”
Machine learning offers this means. Using cognitive computing, software programs can identify key words in texts and transcripts of voice recordings that cannot be captured in a database or spreadsheet. What previously escaped the lens of claims management is now in view. Even better, the models’ powerful mathematical processes crunch this information along with other data to produce remarkable insights about a particular claim or group of similar claims, helping carriers reduce overall claim costs while giving brokers a better grip of their clients’ loss prevention needs. “We’re not replacing the adjuster, but we’re getting there,” Franzis says.
Gaining Traction
One of the first carriers to seize upon the value of machine learning in reducing workers compensation claim severity is Chubb. A typical workers comp claim comprises an enormous volume of disparate data. This ranges from the actual claim filing to the notes of the claims examiner; the medical bills associated with the illness or injury; the claimant’s demographic information, job tenure and medical history; and the different healthcare services, medications and physical therapy prescribed for the claimant. This information is compiled on a continuous basis over time. An example is the ongoing progress reports that claims adjusters issue.
Using advanced analytics, Chubb, which recently merged with Ace, is analyzing this cumulative data in real time. Called Chubb 4D, the model amasses and analyzes all the information cited above, as well as other data typically attached to a claim file, such as police reports and litigation documents.
“Using 4D, a claims supervisor can learn how many times the word ‘pain,’ for instance, appears in the claims notes by the adjuster,” says Scott Henck, a senior vice president at Chubb. “If the word appears more than a predefined number of times, it may trigger the need for deeper analysis and possible action.”
The cognitive computing system also can discern possible fraudulent activities by third parties and identify previously unknown factors affecting potential claims severity. In either case, the information would direct claims supervisors to investigate the situation and take actions to mitigate the causes of loss.
Each time 4D projects a certain claim outcome, it becomes part of the system’s knowledge base, assisting more refined predictions in the future. In other words, each action taken to reduce a claim’s severity is additional grist for the mill, a finding that adds to other information to improve overall prediction accuracy. “We’re now turning our predictive analytics into prescriptive analytics, providing claims professionals with specific information drawn from the predictions to do their job better,” Henck says.
As an example where analytics has reduced claim severity, Henck cites the case of an electrician who fell from a ladder, resulting in the fracture of four lumbar vertebrae. Based on the specifics of the injury and the employee’s demographic and other factors, 4D prescribed a variety of claim actions. These included a visit to the emergency room within 24 hours, immediate contact with the insured and a quick referral to a nurse resource. Each action led to subsequent actions, such as an early determination of the person’s availability for modified work. All these factors combined to reduce the overall claim costs, when compared to similar claims historically, by more than $46,000.
It sounds almost too good to be true, and it is to the extent that machine learning is not a replacement for human decision making. “We don’t say to our claims specialists, ‘This is what you have to do with this claim.’ We explain instead that the model indicates this particular type of claim is likely to have unexpectedly high severity and in such cases the following actions are useful to take,” Henck says. “We want them to apply their own expertise to this information to drive the best outcome.”
Working Wonders with Watson
Swiss Re’s cognitive computing partnership with IBM Watson is predicated on the reinsurer’s life and health business. Last fall, the massive carrier announced the first of a planned series of projects with IBM Watson utilizing the tech company’s now-famous cognitive computing system Watson. Named for Thomas J. Watson, IBM’s first chief executive, the computer’s vast storehouse of knowledge is seen as a way to widen Swiss Re’s profit margins in the extremely tight reinsurance business.
“We have always considered ourselves to be a knowledge-based company, but it had become clear to us that the industry was undergoing a major digital analytic revolution,” says Riccardo Baron, big-data and smart-analytics lead in the Americas division of Swiss Re.
To compete successfully, insurers and reinsurers need to identify emerging risks and loss trends better than they have in past, spotting operational issues and opportunities at a higher frequency than done before with traditional analytics. “We decided we needed to become more of a data-driven company, transforming areas of
our business that are essentially data-supported into being really data-driven,” Baron says.
This transformation is under way with the first application of IBM Watson’s cognitive computing technology platform to assist Swiss Re’s life and health reinsurance business. “No human could browse millions of articles in scientific and medical journals to learn the best treatments for a particular disease,” Baron says. “Since all of us are genetically different, these treatments may differ. In the same way that hospitals are using big data to improve patient outcomes, we are hoping to apply this knowledge to our life and health underwriting decisions.”
To acquire this knowledge, Watson has been configured to acquire specific intelligence from a vast array of data sets. These data include medical treatments, patient outcomes, property risks, demographic changes, man-made and natural disaster criteria, and more. This artificial intelligence connects the dots, informing better underwriting decisions to help achieve Swiss Re’s margin-widening objectives. The project is still in the R&D stage, Baron says, with Phase One recently concluded. “Nevertheless, we’ve already identified other areas of our business to apply cognitive computing to next,” he says.
Brokers on the Bandwagon
Not just insurance and reinsurance carriers are taking advantage of the predictive powers of machine learning systems. Willis Towers Watson, for example, is applying artificial intelligence to its claims processing, searching for unusual event correlations that might affect expected outcomes. A case in point is the global brokerage’s directors and officers liability insurance book of business. “The underwriting community is pretty well established in understanding that two non-correlated factors—market capitalization and stock price movements—in fact do correlate with D&O exposures,” says Ben Fidlow, the firm’s global head of core analytics. “We wanted to find out what else might be out there.”
The goal was to identify other seemingly uncorrelated data and ferret out their respective impact on D&O claims frequency and severity. Using machine learning, the brokerage compared the impact of different equity, fixed income and hedge fund indexes published by MSCI with claim movements, discerning a correlation. It did the same with rating data provided by accounting and audit firms, which would appear to be more of a stretch. But this was not the case. “Both data sets have been shown to have significant predictive accuracy,” Fidlow says.
Other reliable indicators of D&O claim outcomes include actions taken by activist investors and hedge funds that take a short interest in a stock or fund. The devil is in the details, as not all moves by activist investors or hedge funds will affect the claim outcome.
“The challenge always is weighting the various factors,” Fidlow explains. “It requires a combination of stringent mathematical manipulations and human knowledge of the risk. It’s a delicate balance. One must not blindly follow the math, no matter how smart it is.”
Why is Willis Towers Watson interested in D&O claim outcomes?
“Our job is to present the most accurate risk profile of a client to insurers,” Fidlow replies. “When an insurer looks at a huge portfolio of risk, it’s hard to see the forest for the trees. We’re closer to the client and their data. Using predictive analytics, we can provide more accurate assumptions of their true risk.”
Aon also is leveraging machine learning, in its case as part of an exploratory mission to better determine and serve the needs of its clients on an industry-by-industry basis. “It’s important for us to understand the exogenous factors that may be at play in an industry,” explains Todd Curry, CEO of the Aon Centre for Innovation and Analytics. “We have a team that slurps data on all the newsfeeds that we can get.”
This external macroeconomic and geopolitical information is fed into the brokerage’s predictive analytics models to inform us as to which products and services best suit a particular client. A tightening market in an industry might require revised policy coverage limits, terms and conditions to maintain the client’s business. “We’re still in our formative stages analyzing these factors, but all signs indicate that this is highly useful information,” Curry says. “Our goal here is to put these tools in the hands of our people worldwide to create a network effect driving expanded use of analytics.”
Many organizations have increased their commitment to investing further in machine learning tools and in the recruitment and hiring of employees with more sophisticated technological skill sets. As Curry put it, “The industry has been slow to hire people with analytical talents. It’s now clear that this has come up. That’s a very good thing.”
Machines may become wiser than people, but people can become wiser, too.