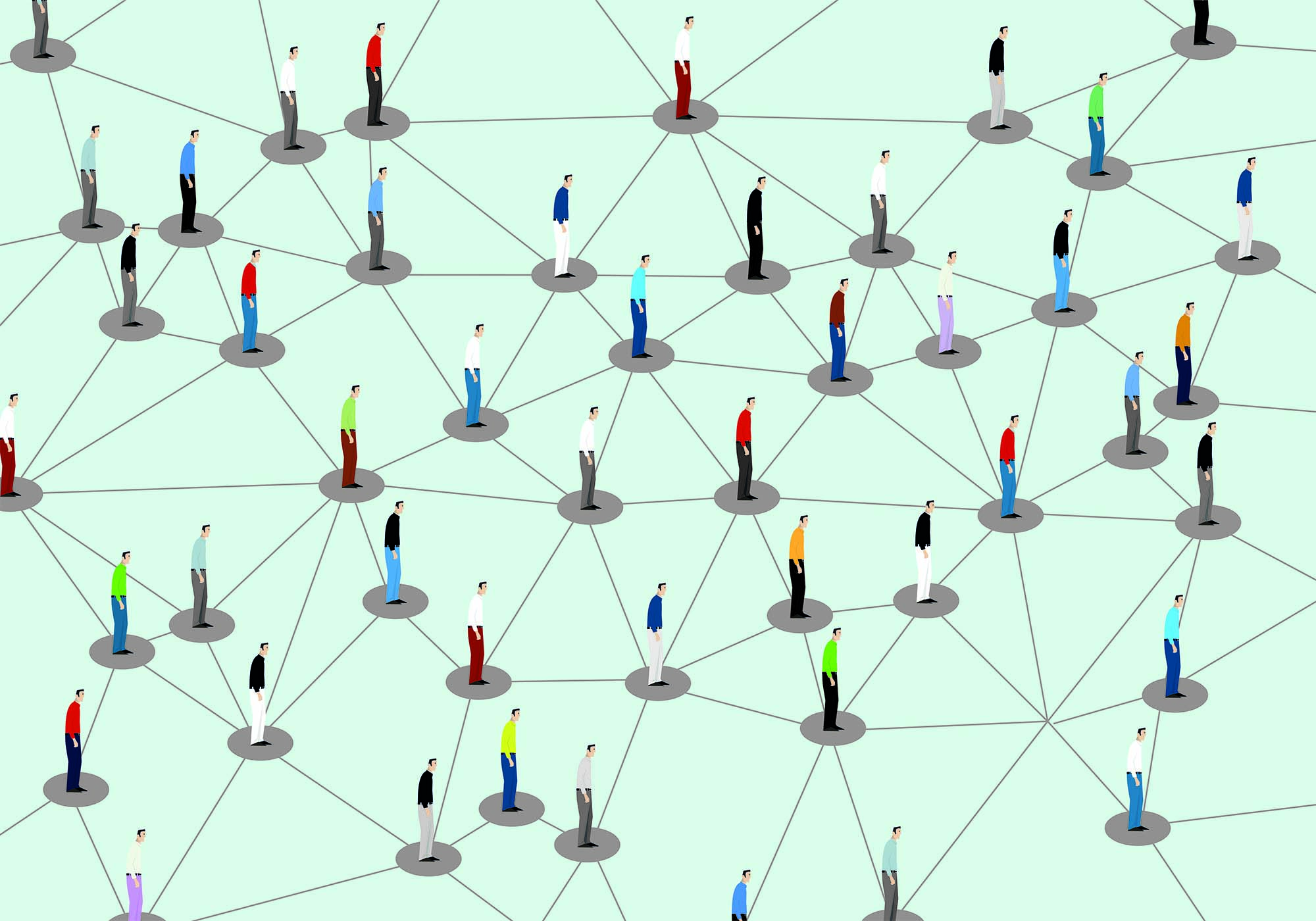
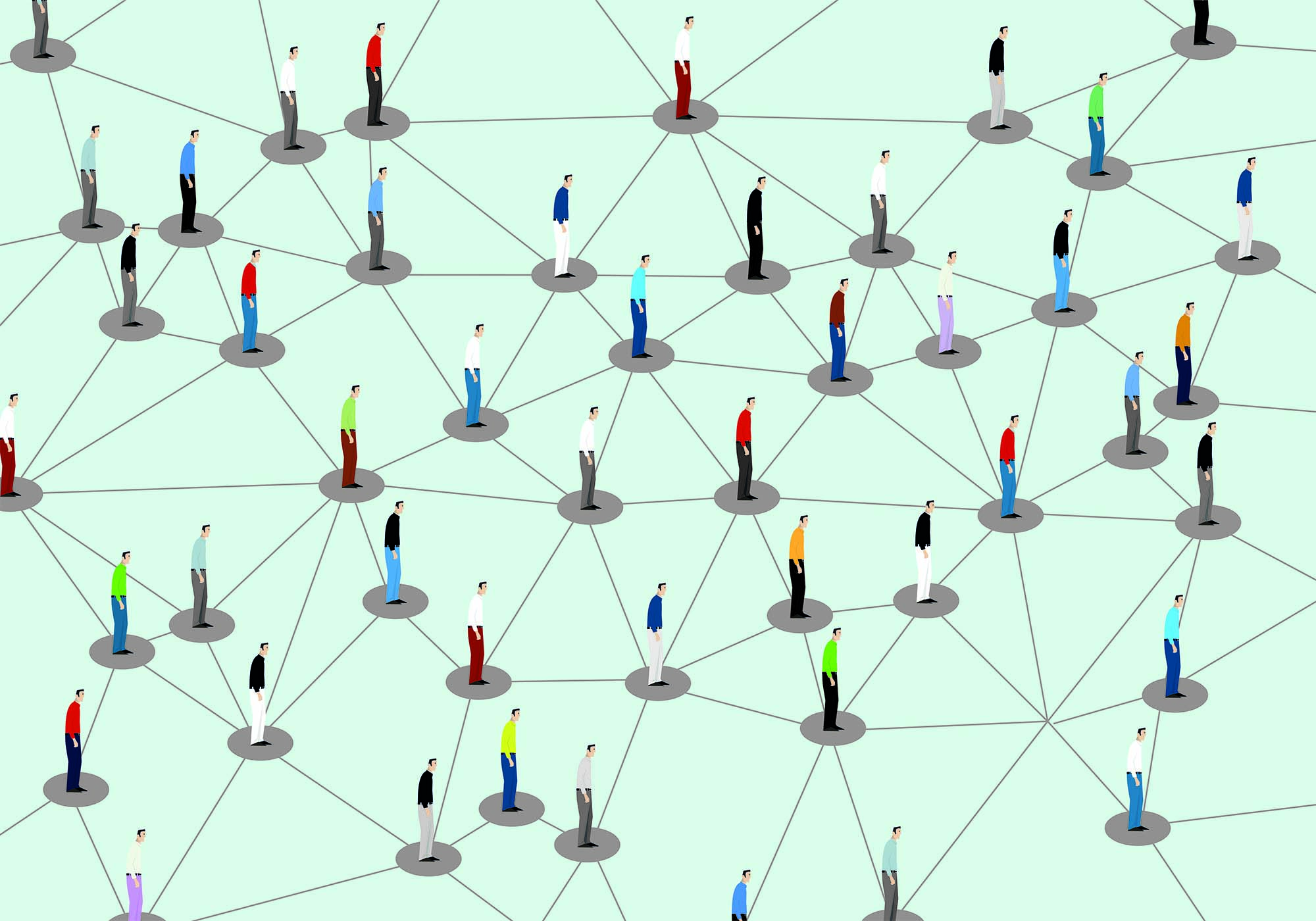
Scott MacEwen, SVP of Consulting at Deerwalk, a data management and healthcare analytics software company, sat down with Leader’s Edge to discuss the key to managing population health and why it’s about much more than delivering care.
As more research becomes available, it’s becoming evident that SDOH may play an even greater role in population health than the actual care that’s being delivered. While analyzing commercial populations, our data scientists found strong correlations between the claims data and SDOH, not only with regards to WHERE people were getting their care, but what KIND of care they were getting. For example, the data suggests that some individuals with less access to care are more likely to take advantage of alternative care models if they’re given the option. It’s apparent that in addition to focusing on the cost and quality of care, employers can benefit from incorporating SDOH into population health analysis. This will help them better gauge the incidence and prevalence of disease in their population, member behavior when it comes to things like the utilization of services and adherence to care recommendations, and program participation and the effectiveness of certain interventions.
We recently integrated a new data set that reveals correlations between demographics and health outcomes without the use of claims data called the “Area Deprivation Index”(ADI). ADI ranks neighborhoods by relative socioeconomic status in a specific region and can be used to stratify members based on their zip code. Our analysis indicated a consistent relationship between socioeconomic status and the use of preventative services. For example, a member residing in an area of relative socioeconomic disadvantage is more likely to encounter barriers while trying to access care, making them less likely to use preventative services and more likely to end up visiting the emergency room. Lack of preventative care also increases the likelihood that a potentially preventable disease will go undiagnosed, leading to worsening health status or other co-morbid conditions.
Access to actionable information from machine learning models and reporting tools that integrate SDOH with claims data can help employers better assess and plan for the healthcare utilization of their populations. This type of analysis can guide informed decisions around plan design (e.g., adjusting ER co-pays, adding telemedicine benefits), outreach programs (e.g., adding a health navigator benefit or conducting targeted educational campaigns), and other initiatives (e.g., contracting transportation services to improve access) to alleviate socioeconomic disparities and better serve underserved populations.
Breaking it down a bit further, Deerwalk’s data scientists used a statistical measure to look at the relationship between ADI scores and member utilization events for a commercial population. The results showed a strong correlation between ADI scores and emergency room (ER) visits, office visits, and urgent care visits. Members with high ADI scores (at a relative socioeconomic disadvantage) also had higher ER usage and fewer office and urgent care visits. This suggests that plan design does not always account for those members that may be at a significant disadvantage when it comes to utilizing certain benefits.
To better assess cost-sharing implications for a population subset, an employer could use identification and stratification analysis tools to categorize members by demographics, such as zip code. They could then use an actuarial-based plan design model to run different scenarios to see how changes to co-pays, co-insurance, and deductibles could impact plan and member cost-sharing and incentivize member behavior.
Achieving interoperability requires organizations to first get a handle on their data (this process is sometimes referred to as “master data management”). In the healthcare analytics space, most large employers essentially “own” or have the rights to their data. However, when it comes to collecting and integrating that data into their systems, reliance on outside vendors (e.g., PBMs, insurers, TPAs, etc.) to deliver data files can sometimes make this a lengthy and complicated process. In addition to challenges in data retrieval, certain system and process limitations (such as platform architecture) can make it difficult to ensure all stakeholders have convenient access to clean, accurate, up-to-date data.
With this in mind, it’s apparent that both data management and interoperability are key when it comes to effectively managing the health of a population. Not being able to connect the dots across various data types means missing out on valuable insights that could help identify exactly where to make that small tweak to achieve ROI for a program that was previously suffering or allocate resources in a totally new direction.
With COVID-19, we knew our clients would look to us as they prepared to pivot quickly and adjust their strategies to approach this uncharted landscape. We rolled out a series of webinars, dashboards, and companion guides to address client questions and anticipate concerns around future cost and utilization. We designed our approach in a way that allowed our clients to map and reroute their strategies as time lapsed and the pandemic introduced new challenges, offering guidance along the way with tools and resources to:
1. Identify particularly vulnerable segments of their populations and assess critical areas of exposure,
2. Model and prepare for the potential costs and plan experience during COVID-19,
3. Track and measure the actualized all-in costs of the pandemic through COVID-19-specific diagnosis and testing codes, and
4. Implement recommendations around health and safety concerns for employees who continue to work or will soon be returning to work.
We also brought in multiple experts, including physicians, an actuarial consultant, and the leader of an onsite clinic organization to add some color commentary with real world advice on how to best manage during this crisis.